ChatGPT-4 Rivals Human Financial Analysts, Says Study
Gen AI might just be able to help you play the stock market if you can tune a GPT the correct way.
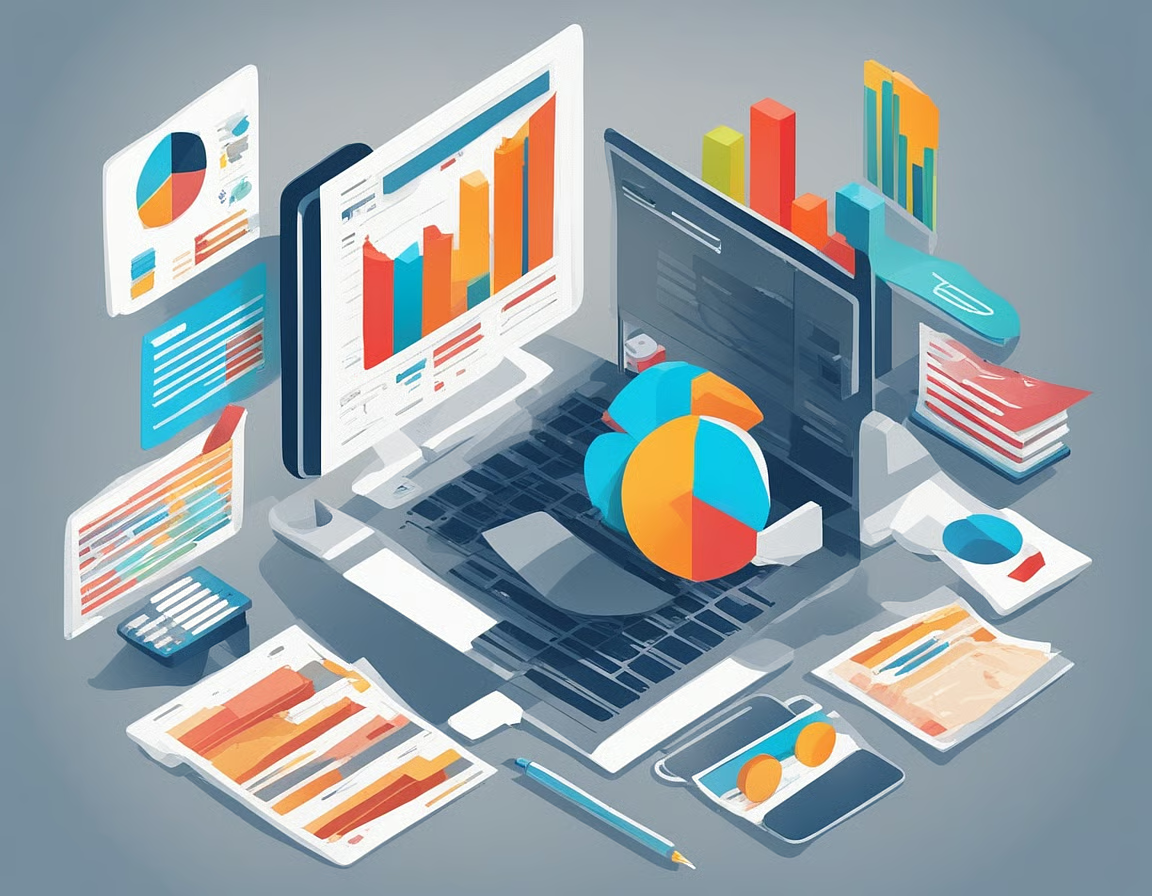
Artificial intelligence has been a part of the financial markets for a while. Wire agencies like Bloomberg and Reuters already have their own specialised models to track movement in the stock markets across the world, as well as exchange filings that companies make to stock exchanges.
Earlier in January this year, Bloomberg released a generative AI update for its terminals that summarises earnings and analyses the financial performance of companies.
But if research from the University of Chicage’s Booth School of Business is to be believed, OpenAI’s ChatGPT-4 Turbo (Generative Pre-trained Transformer-4) can do all of that at the same level as specialised large language models and sometimes even better.
Teaching GPT-4 Financial Analysis
Given that financial statement analysis requires both qualitative and quantitative data, earnings predictions are often challenging, even for specialised LLMs.
First, the researchers anonymised financial statements and then asked the LLM to “analyse the two financial statements of a company and determine the direction of future earnings.”
Second, in an effort to get GPT-4 to replicate the way human analysts come to predictions for earnings, the Booth researchers used chain-of-thought prompts.
In these types of "prompts," when giving instructions to an LLM, you encourage it to not only give you the answer but also explain the steps it took to get there. To put it simply, think of a math teacher expecting you to show the steps you took to get the correct answer or they won’t give you full marks.
Because an LLM doesn’t have the ability to reason and perform judgement like a human, the researchers gave GPT-4 the following prompts:
Assume the role of a financial analyst, whose job it is to perform financial analysis.
Take note of all significant changes in certain financial statement items, like big changes in revenue, profit, etc.
Compute key financial ratios while also explaining the formula used for the calculations and how it produced specific numbers. These financial ratios are used to identify the financial health of a company.
Once the calculation of ratios is complete, GPT-4 has to interpret what the numbers mean.
Using all the information received from the previous steps, identify whether the company’s earnings will go up or down in the next period.
To conclude, the model must then provide an explanation of its predictions so that the researchers can see the thought process of GPT-4.
The research shows that by using a CoT prompt, the methodology of the study was ingrained into the model, which helped in “guiding it to mimic human-like reasoning in its analysis”.
Of course, the most important question is how did the LLM perform?
How Did GPT-4 Perform?
Turns out, it did a pretty good job!
The researchers showed that, through a simple prompt, GPT-4 achieved an accuracy of 52%. While that may seem low, the accuracy of predictions from a human analyst is at 53% for the first month, and the number climbs to 56% and 57% for three- and six-month forecasts, respectively, given that they incorporate more timely information.
However, by prompting GPT-4 through CoT, the performance improved significantly, with the LLM achieving 60.31% accuracy. This is very close and nearly on par with specialised artificial neural networks, which have an accuracy of 60.45% and work on the same parameters for calculations.
In some cases, the researchers found that GPT-4 was outperforming these specialised neural networks and was able to pick up the slack where ANNs struggle.
Conclusion
According to the conclusion from the researchers, GPT shows “remarkable aptitude for financial statement analysis and achieves state-of-the-art performance without any specialised training.”
The researchers found that GPT could actually perform a task that typically requires human expertise and judgement if it's just provided with the right data set to look at.
The follow-up question to be asked, of course, is if these kinds of GPTs can replace humans.
Short answer: No.
Long answer: No, but they certainly work well together.
The folks at Booth write that “GPT and human analysts are complementary, rather than substitutes." Further, the research found that LLMs are able to outperform human analysts when it comes to predicting the direction of a company’s future earnings and have a “large advantage” over human analysts when it comes to exhibiting expected bias and disagreement.
The researchers say that an LLM can actually help an analyst when they are underperforming. An analyst, on the other hand, can “add value when additional context, not available to a model, is important.”
In an amusing twist, despite extensive testing, the researchers concluded that understanding the model's predictions remains elusive. They noted that it has been "empirically difficult to pinpoint how and why the model performs well."