Two-Fifths Of Generative AI Solutions Will Be Multimodal By 2027: Gartner
Along with open-source large language models, multimodal gen AI has high-impact potential on organisations within the next five years, Gartner said.
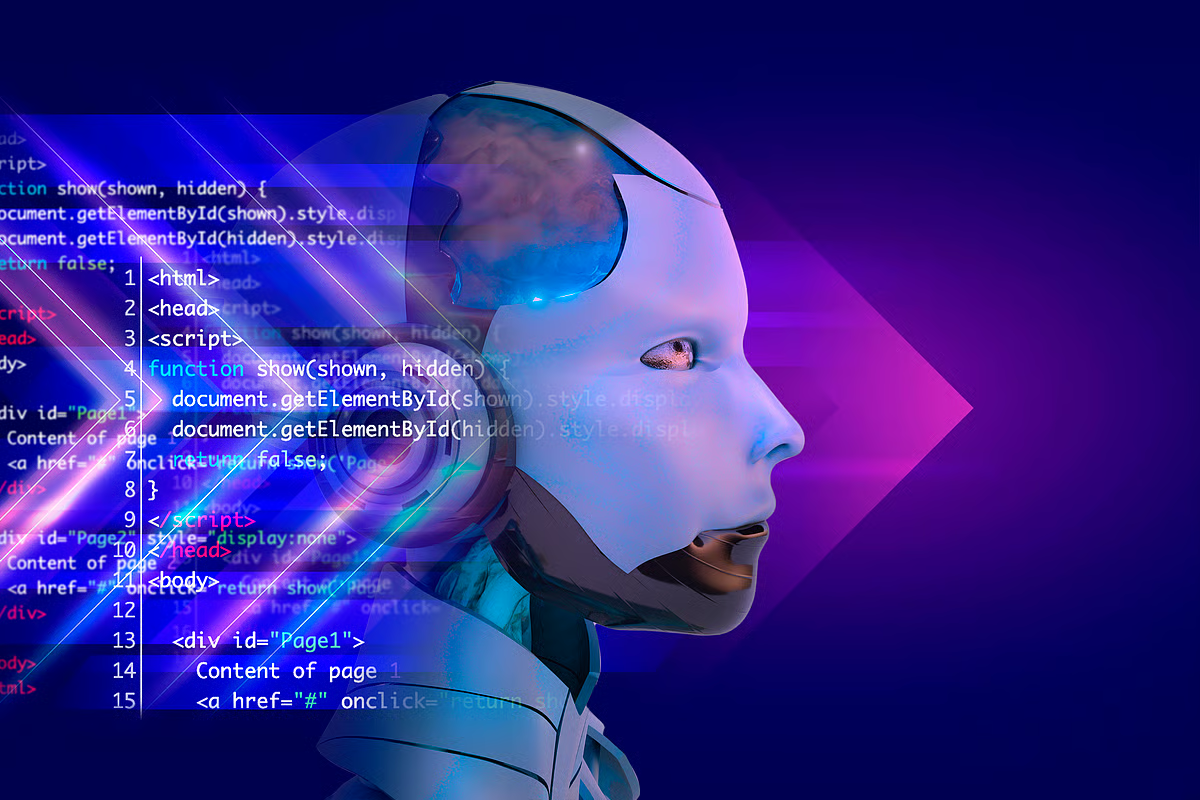
Two-fifths, or 40%, of generative artificial intelligence solutions will be multimodal (text, image, audio and video) by 2027, up from 1% in 2023, according to research and consulting firm Gartner Inc. This shift from individual to multimodal models provides an enhanced human-AI interaction and potential for differentiated generative AI-enabled offerings.
According to Gartner experts, as the gen AI market evolves towards models natively trained on more than one modality, this helps capture relationships between different data streams. It also has the potential to scale the benefits of gen AI across all data types and applications, and allows AI to support humans in performing more tasks.
“Gen AI is in the trough of disillusionment with the beginning of industry consolidation. Real benefits will emerge once the hype subsides, with advances in capabilities likely to come at a rapid pace over the next few years,” said Arun Chandrasekaran, distinguished VP analyst at Gartner.
According to Gartner, along with open-source large language models, multimodal gen AI has high-impact potential on organisations within the next five years.
Multimodal Gen AI
Multimodal gen AI will impact enterprise applications by enabling the addition of new features and functionality. The impact is not limited to specific industries or use cases, and can be applied at any touchpoint between AI and humans.
While currently, many multimodal models are limited to two or three modalities, this is expected to increase over the next few years. For enterprises, early adoption of multimodal gen AI has the potential to lead to competitive advantage and time-to-market benefits.
Multimodal gen AI is important because data is typically multimodal. When single modality models are combined or assembled to support multimodal gen AI applications, it can lead to latency and less accurate results, resulting in a lower quality experience, Gartner experts said.
Open-Source LLMs
Open-source LLMs are deep-learning foundation models that can accelerate enterprise value of gen AI by democratising access and allowing developers to optimise models. They provide access to developer communities in enterprises, academia and other research roles that are working to make the models more valuable.
“Open-source LLMs increase innovation potential through customisation, better control over privacy and security, model transparency, ability to leverage collaborative development, and potential to reduce vendor lock-in,” said Chandrasekaran.
Gartner also expects that domain-specific gen AI models and autonomous agents are technologies with high potential, reaching mainstream adoption within 10 years.
Domain-Specific Gen AI Models
Domain-specific gen AI models are optimised for the needs of specific industries, business functions or tasks. They can improve use-case alignment within the enterprise, while delivering improved accuracy, security and privacy, as well as better contextualised answers. This reduces the need for advanced prompt engineering compared with general-purpose models.
“Domain-specific models can achieve faster time to value, improved performance and enhanced security for AI projects by providing a more advanced starting point for industry-specific tasks,” said Chandrasekaran.
Autonomous Agents
Autonomous agents are combined systems that achieve defined goals without human intervention. They use a variety of AI techniques to identify patterns in their environment, make decisions, invoke a sequence of actions and generate outputs. These agents have the potential to learn from their environment and improve over time, enabling them to handle complex tasks.